Author: Douglas Gantenbein. This article originally appeared at Amazon Science
Finding the optimal route between multiple destinations — the traveling salesman problem — is a challenge regularly faced by Amazon’s Last Mile team. Meeting that challenge has meant developing planning software to allow Amazon’s delivery fleet to find the most efficient routes. But what happens when drivers must deviate from those routes? Drivers have access to real-time information — road blockage, congestion, parking, etc —and other knowledge and know-how that existing optimization models don’t capture.
“Despite the tremendous advances in routing optimization over the last decade, there remains an important gap between periodic route planning and real-time route execution,” said Beryl Tomay, Amazon vice president for Last-Mile Delivery.
That’s why in February Amazon collaborated with MIT’s Center for Transportation & Logistics (MIT CTL) to develop a competition that challenged academic teams to train machine learning models to predict the delivery routes chosen by experienced drivers.
On July 30, the winners of that contest, dubbed the Amazon Last Mile Routing Research Challenge, were announced. “The Last Mile routing challenge is a classic problem,” said Daniel Merchan, a senior research scientist on Amazon’s Last Mile team. “Our participants worked for more than three months to come up with innovative, data-driven solutions.”
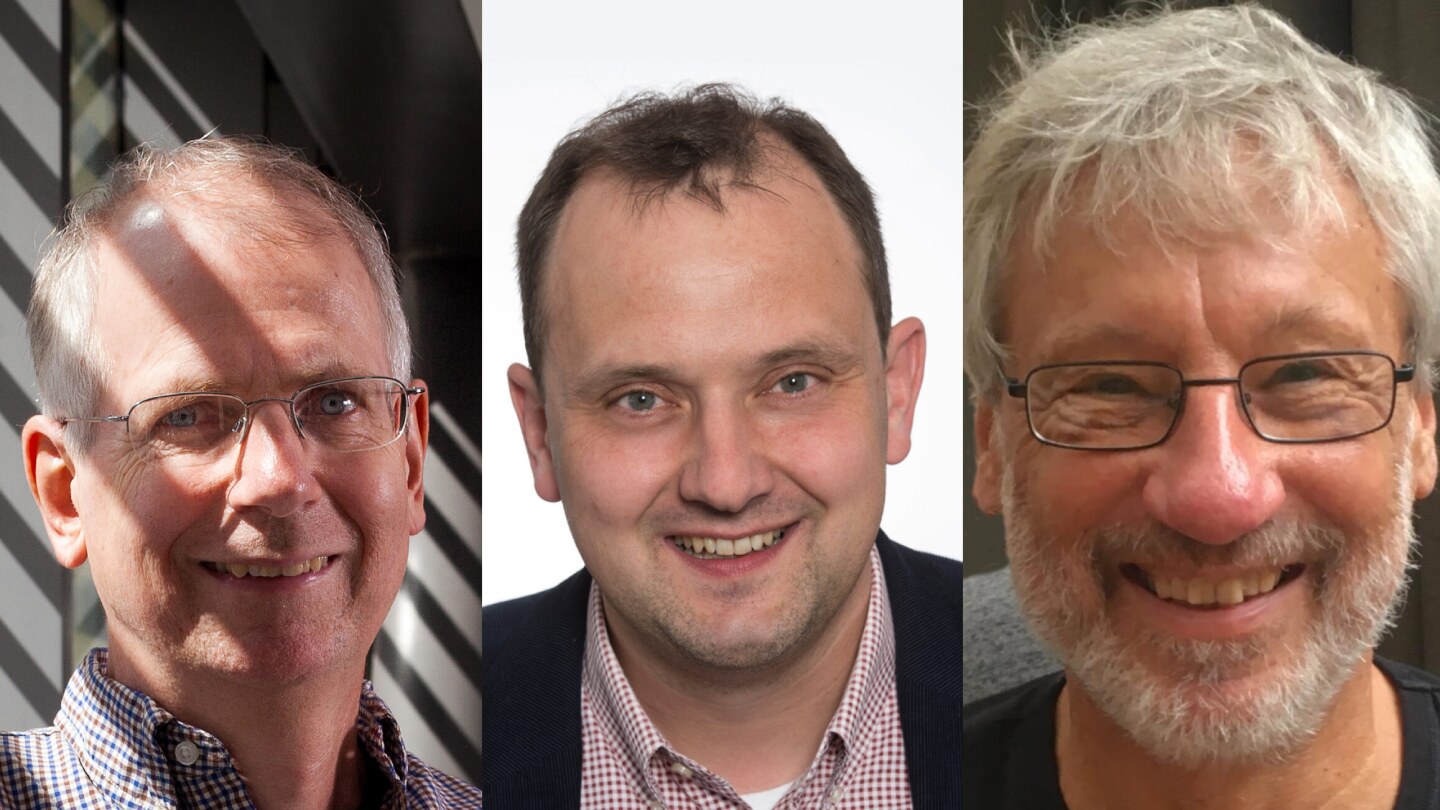
Team Passing Through, comprising scholars from three separate universities, took top honors, winning $100,000. The team’s members are:
William Cook, professor of combinatorics and optimization at the University of Waterloo, Canada; Stephan Held, associate professor with the Research Institute for Discrete Mathematics at the University of Bonn, Germany; and Keld Helsgaun, associate professor emeritus in computer science at Roskilde University, Denmark.
“The challenge was a huge contribution to the research community, providing a massive collection of test instances,” the team said in a statement provided to Amazon Science. “In a single post, Amazon made publicly available more real-world examples of the traveling salesman problem than had been collected in total over the past 70 years.”
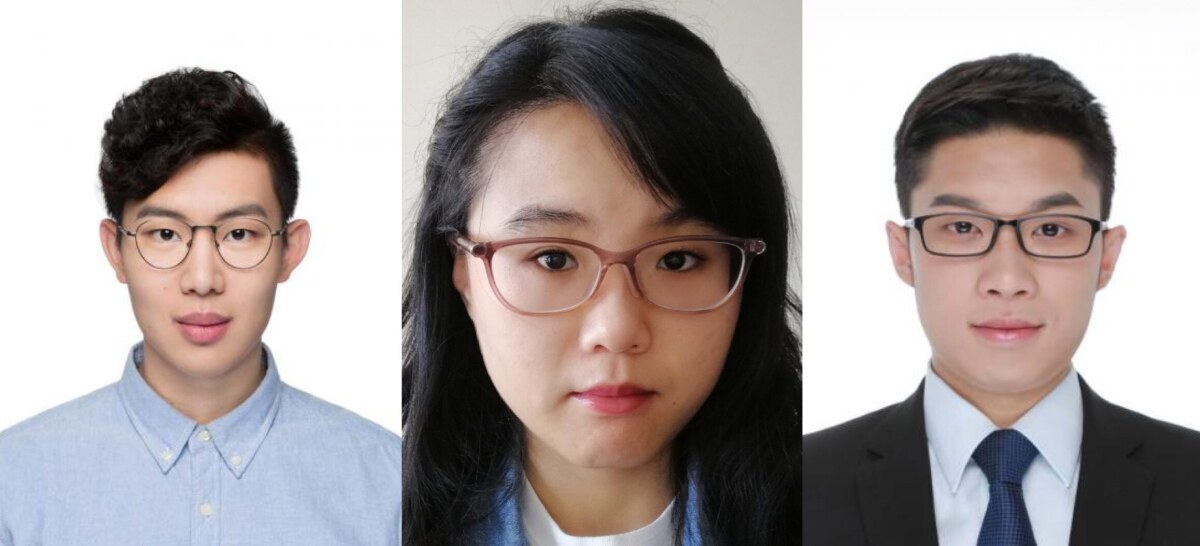
The $50,000 second-place prize went to Team Permission Denied, comprising a trio of MIT Ph.D. students — Xiaotong Guo, Qingyi Wang, and Baichuan Mo — while Team Sky is the Limit, comprising Okan Arslan, assistant professor at HEC Montreal, and Rasit Abay, a Ph.D. student at the University of New South Wales Canberra, won the $25,000 third-place prize.
More than 220 teams participated in the competition, with 45 competing in the final round. Overall, the teams represented 71 different universities and 22 countries. Entrants ranged in academic level from undergraduate to retired faculty.
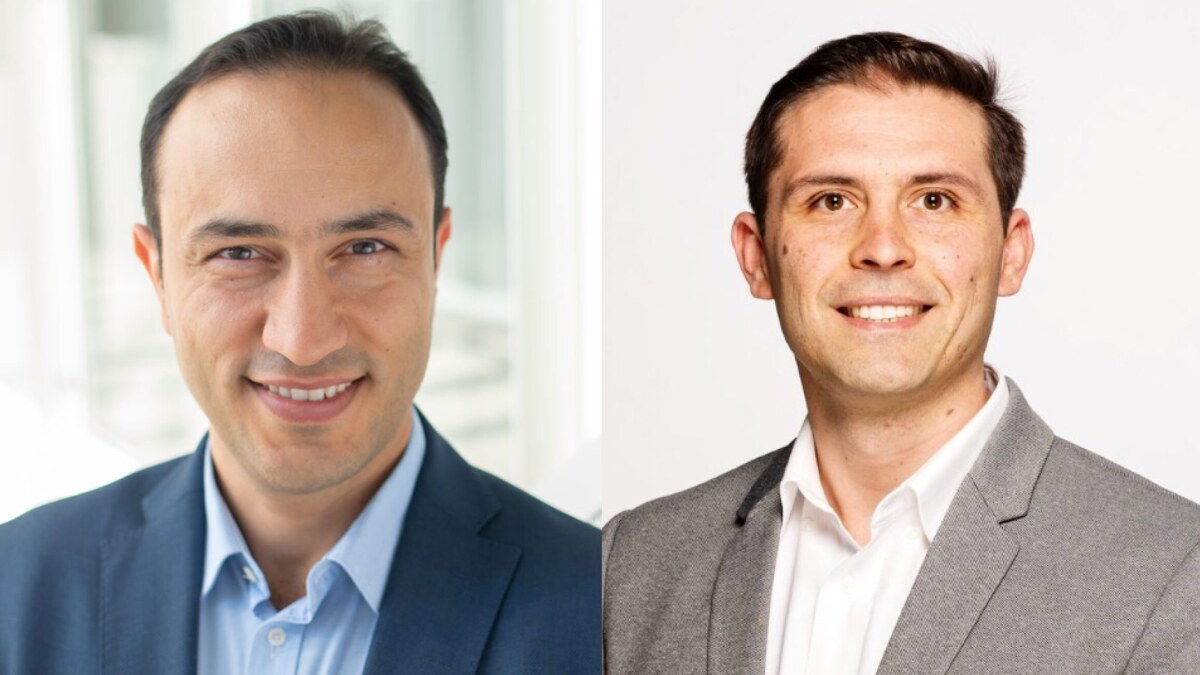
Entrants were given 6,100 historical route records from five areas across the United States to use as a baseline for their project. They also were given more than 3,000 traces of driver-determined routes. Both datasets included driver knowledge. The initial dataset was used for training and testing the model, while the second dataset was utilized for evaluation using both sources of information. Contestants endeavored to build models that could identify and predict drivers’ deviations from routes computed in the traditional manner.
Participants utilized a variety of approaches, including conventional optimization models (some of them enhanced with machine learning components), and wrote short technical papers explaining their approach.
Last Mile Challenge finalists will have an opportunity to publish their research in Transportation Science.
Call for papers A special issue of Transportation Science will be published in conjunction with the Last Mile Challenge. Karthik Konduri, a senior research scientist on the Last Mile team, and Julian Pachon, director and chief scientist for the Last Mile science team, will be co-editors for this special issue. The deadline for submissions is Nov. 30, 2021.